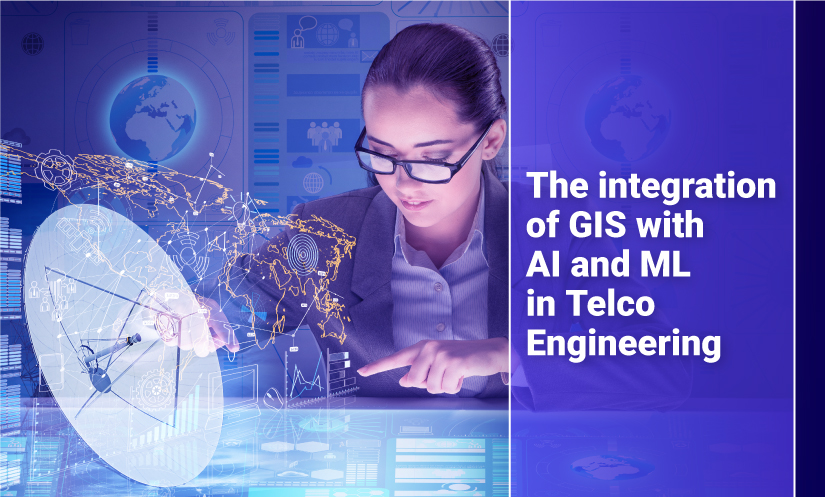
Maps, once basic sketches, have transformed into sophisticated data tools. They’re now used to identify problems, propose solutions and even predict future challenges. Recent advancements have enabled maps to analyse, forecast, and provide valuable insights.
For example, with its extensive network infrastructure exposed to climate risks, AT&T, a leading telecommunications company, employs special mapping tools to mitigate the impacts of hurricanes and other extreme weather events. During a recent major hurricane, the company used satellite imagery integrated with advanced tools to assess damage and prioritize restoration efforts, ensuring critical services remain operational. As climate change intensifies, AT&T forecasts a 5% increase in surface water accumulation by 2050 in the US southeastern regions, prompting proactive planning for network resilience.
The question is, can the same technology revolutionise telecom mapping for similar other critical needs and result in significant cost savings? Let’s find out.
Bridging the coverage gap: A telecom provider’s dilemma
Telecom networks face increasing strain due to surging demand for high-speed services driven by 5G, IoT, and other emerging technologies. The projected growth in connected devices, from 43 billion in 2020 to 51.9 billion by 2025, underscores the urgent need for telcos to optimise network operations and embrace new technologies for planning, design, construction and management.
Efficient network mapping is essential to visualise cost-effective strategies for expanding and upgrading networks, especially in challenging terrains. By understanding geographic and demographic data, telecom providers can prioritise investments where they will yield the highest return.
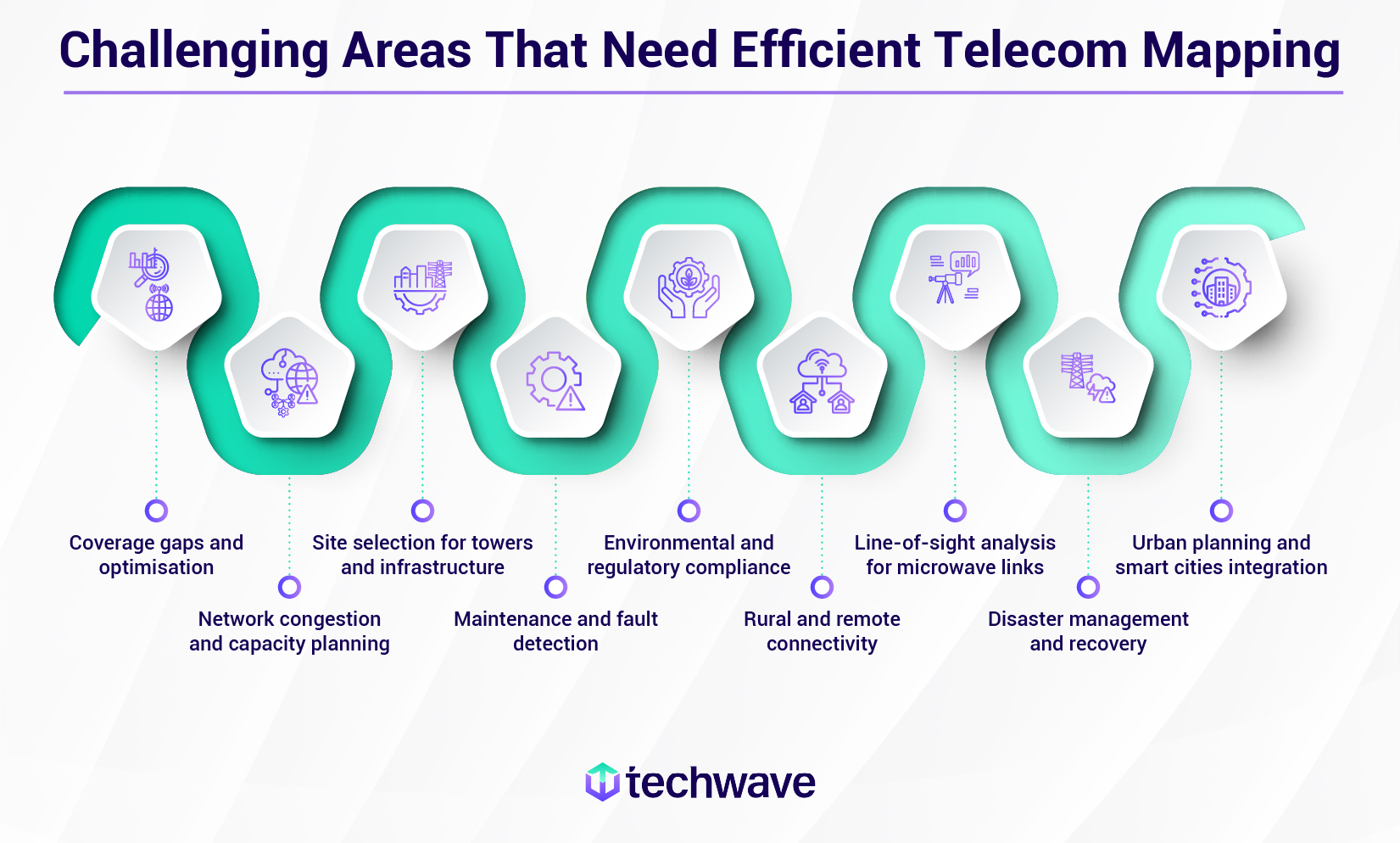
Despite employing Geographic Information System (GIS) mapping software and other tools, that’s a lot of challenges! It’s time for telecom providers to rethink strategies and invest in advanced technologies that can automate processes, improve efficiency, and deliver a superior customer experience.
Integrating AI and ML into GIS: A synergistic approach
A decade ago, the mapping precision we see today with GIS would have been unimaginable. While GIS helps telecom providers tackle challenges to some extent, Geospatial Artificial Intelligence (GeoAI) takes it to the next level. McKinsey’s research indicates that AI could majorly impact telco operations, as field and service activities represent a substantial portion (60-70%) of their budgets.
Imagine telecom providers mapping Europe’s network to pinpoint connectivity gaps. This scenario showcases the limitations of traditional GIS and the transformative power of GeoAI.
GIS empowers providers to map existing infrastructure and identify areas with missing coverage. It also helps pinpoint gaps that affect people. How? Assessing demand by layering data like population density and smartphone usage onto maps to visualise where towers are lacking and guide strategic investments. Sounds good!
However, while GIS helps identify areas with insufficient towers, it falls short in providing specific metrics like the exact number of towers needed and their optimal spacing for reliable coverage.
This is where GeoAI excels. By combining spatial analysis with AI, GeoAI can generate these maps and critical metrics in minutes, delivering insights with unprecedented speed and precision!
Applications of integrating GIS with AI and ML in telco engineering
By leveraging AI’s predictive capabilities and ML’s ability to learn and adapt, GeoAI can address complex challenges such as network planning, optimisation, and customer sentiment analysis. This is particularly crucial for 5G network readiness, as deploying new infrastructure requires a deep understanding of network dynamics.
LiDAR, a specialised branch of GIS, complements GeoAI by providing detailed subsurface mapping. This information helps identify ideal locations for new towers, optimise deployment and minimise costs. Additionally, GeoAI’s ability to analyse vast amounts of imagery from satellites, drones, and aircraft enables telcos to quickly understand rapid changes, calculate metrics that intimidate human capabilities and provide answers to not just “where” but “where now”!
Here are a few other applications in telco engineering resulting from the combination of GIS with AI/ML:
1. Streamlines training data for machine learning in GIS applications
The vast amount of geospatial data from remote sensing systems like drones and LiDAR is too overwhelming for humans to analyse efficiently. AI/ML integrated with drones and LiDAR automates data annotation and labelling, transforming how we process and utilise this data.
- Automated image classification and labelling: With sufficient training, ML algorithms can quickly and accurately identify features in satellite or aerial images—like cities, roads, vegetation, and structures—automating tasks such as infrastructure mapping and land cover classification (distinguishing towers from buildings and tree canopies, etc.) without human intervention.
- Image segmentation and clustering: ML algorithms segment unlabelled data by characteristics and location, creating interactive maps that can reveal valuable insights. For example, areas with dense urban environments or mountainous terrain may present challenges for signal propagation and network capacity. ML algorithms can segment data based on these factors to identify areas with potential coverage gaps or revenue-making opportunities.
- Simulation-based predictions: ML uses historical data to analyse spatial patterns, run simulations and provide accurate predictions, enabling data-driven decision-making for resource planning and management. Telecom companies can use this data (like population density, land use, and existing infrastructure) to optimise placement of network infrastructure, identifying suitable locations for base stations, data centres, and other network components.
In short, AI and ML are making geospatial data analysis faster, more accurate, and more insightful than ever before.
2. Facilitates GeoAI-powered network inventory for telecoms
Telecommunication networks generate massive amounts of data, making effective management critical for maintaining reliable and efficient infrastructure. Traditional inventory methods often lead to manual errors, inaccuracies, and costly setbacks. To overcome these challenges, telecom companies need GeoAI-driven network inventory systems that offer:
- Unified network view: GeoAI provides a comprehensive, up-to-date geospatial view and tracks the location of network assets like towers, BTS, microwave antennas, fibre optic cables, etc. This information can be used to prevent theft, vandalism, or damage from natural disasters.
- Streamlined operations: Automates inventory-related tasks, reduces manual errors, and boosts operational efficiency.
- Optimised design & planning: Speeds up the inventory design or mapping process, enhances network performance, and reduces costs.
- Field integration: Ensures accurate, real-time communication between field teams and planners by providing real-time data updates, precise asset tracking and a centralised data repository.
This boosts overall design productivity, delivers compliant, cost-efficient network plans and cuts construction costs.
3. Incorporates 3D technology into GeoAI modelling
As a telecom provider, do you feel stuck in a never-ending loop of challenges, always playing catch-up?
Enter AI-powered 3D modelling and digital twins—highly accurate, dynamic replicas of real-world environments. These tools can rapidly transform geospatial imagery into detailed, expert-level maps, driving data-driven decisions across industries.
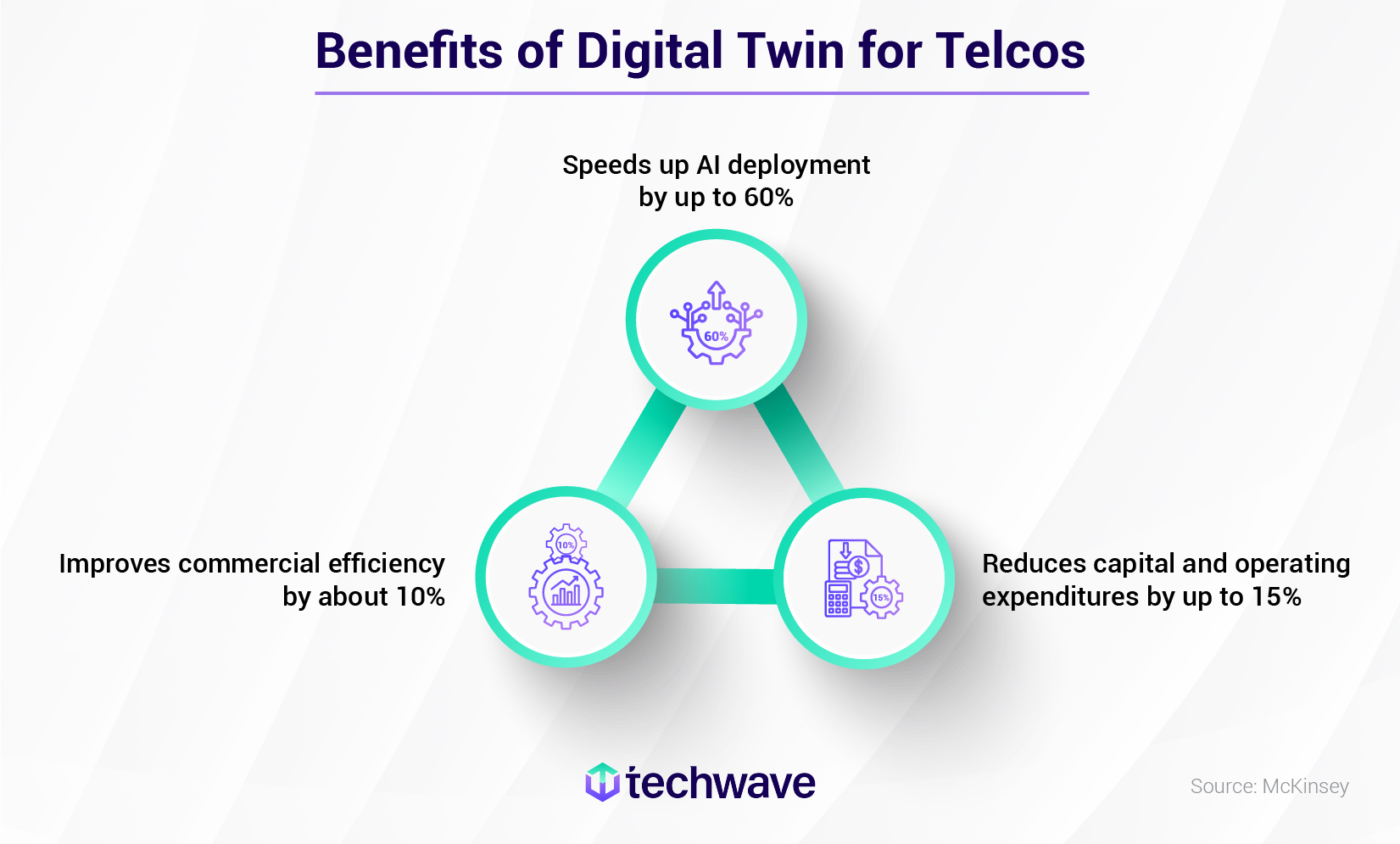
Benefits of Digital Twin for Telcos
- Speeds up AI deployment by up to 60%
- Reduces capital and operating expenditures by up to 15%
- Improves commercial efficiency by about 10%
Understanding and leveraging digital twins is no longer optional for the telecom industry—it’s critical for staying ahead in an ever-evolving landscape. They are fast becoming the go-to solution for major telecom players.
By creating virtual models of real-world telecom infrastructure, digital twins enable unparalleled data analysis, scenario modelling, and predictive capabilities. For instance, ArcGIS (a product developed by Esri, one of the world’s leading suppliers of GIS software) leverages the digital twin technology to accurately model entire networks, capturing fibre-optic, coaxial, wireless, and hybrid systems in precise 2D and 3D formats, while ensuring alignment with industry standards and best practices.
4. Integrates computer vision with GeoAI
AI and computer vision automate tasks that were once done manually. Deep learning leverages Convolutional Neural Networks (CNNs), an artificial neural network specifically designed for processing image data, to dramatically improve computer vision’s ability to process and understand visual information. This breakthrough has opened up new possibilities for automated tasks in telco engineering.
Here’s how deep learning with computer vision tackles these massive datasets:
- Image classification: CNN categorises images like humans into different categories, such as land cover types, buildings, and vehicles.
- Object detection: Deep learning models can detect and localise objects in imagery, crucial for GIS tasks like infrastructure mapping and anomaly detection.
- Semantic segmentation: This technique labels each pixel by category (e.g., building, tree, road) but treats objects of the same type as a single entity, without distinguishing between individual instances. For example, multiple buildings are all labeled “building,” without identifying each one separately.
- Instance segmentation: Instance segmentation is a step ahead of the semantic technique as it improves image analysis by classifying each pixel and identifying individual instances within the same object category, such as labeling each building separately. This precision is vital for recognizing or counting unique assets like cell towers.
AI’s ability to “see” empowers GIS analysis, unlocking insights previously hidden under the vast amount of geospatial data. For example, all the above are powerful techniques for asset condition assessment and remedial actions. CNNs can assess the overall health of assets by classifying them as “good” or “damaged,” while object detection can pinpoint specific issues like corrosion or breakage. Semantic segmentation helps identify broader areas requiring attention, such as vegetation near cell towers, aiding in risk assessment and preventive maintenance. However, for precise asset-level analysis and targeted remedial actions, instance segmentation is crucial. By identifying and labelling individual instances within object categories, such as individual cell towers, instance segmentation enables detailed monitoring, early detection of wear and tear, and efficient prioritisation of repairs and replacements. This level of precision significantly improves telecom asset reliability, reduces downtime and enhances overall operational efficiency.
5. GeoAI-driven Augmented Reality (AR)
Augmented reality overlays digital information onto the real world, enhancing users’ perception of their environment. When combined, AR and GeoAI can create powerful applications such as interactive maps, virtual tours, field operations and urban planning.
For example, a telecom technician is tasked with diagnosing a complex network outage in a remote area exposed to a high-risk operating environment. Traditional troubleshooting methods would involve sending a field worker to the site, potentially leading to significant delays, related costs and safety concerns. Because, despite strict safety protocols and years of experience, workers often overlook and fail to respond to everyday hazards. By leveraging GeoAI-driven AR solution:
- The field worker can connect with a remote telecom expert using AR apps on smartphones and tablets. The remote expert can overlay digital annotations, diagrams, and instructions directly onto the worker’s field of view, providing real-time guidance on troubleshooting steps.
- The field worker can interact with the remote expert using voice commands or gestures, allowing seamless collaboration without interrupting their work.
So, AR-enabled remote assistance can reduce downtime, minimise the need for on-site visits, and speed up network repairs. By reducing the number of truck rolls and on-site technician visits, telecom companies can significantly cut costs associated with equipment downtime and travel expenses!
Techwave: One-stop solution to all your telecom engineering needs
Tired of struggling to expand your reach and deliver cutting-edge telecom solutions? At Techwave, we help telecom service providers overcome these challenges and achieve their goals. As a leading AI and ML provider, we help businesses innovate and excel by driving operational excellence, optimizing decision-making, streamlining processes, and elevating customer experiences through Techwave’s FASTRACK AI offerings:
- Experiment as a Service
- AI Strategy Consulting
- Demonstrative AI Prototype
- AI Enablement and Adoption
We are the one-stop shop for all your telecom needs. Our end-to-end network design solutions are tailored to your specific needs. From wireline to wireless, we provide expert guidance and support throughout the process, ensuring seamless, global communication.
Our expertise in field services streamlines your operations, cutting costs and ensuring business continuity. We also offer specialised solutions and services in data & analytics, AI/ML, LiDAR and cloud engineering.
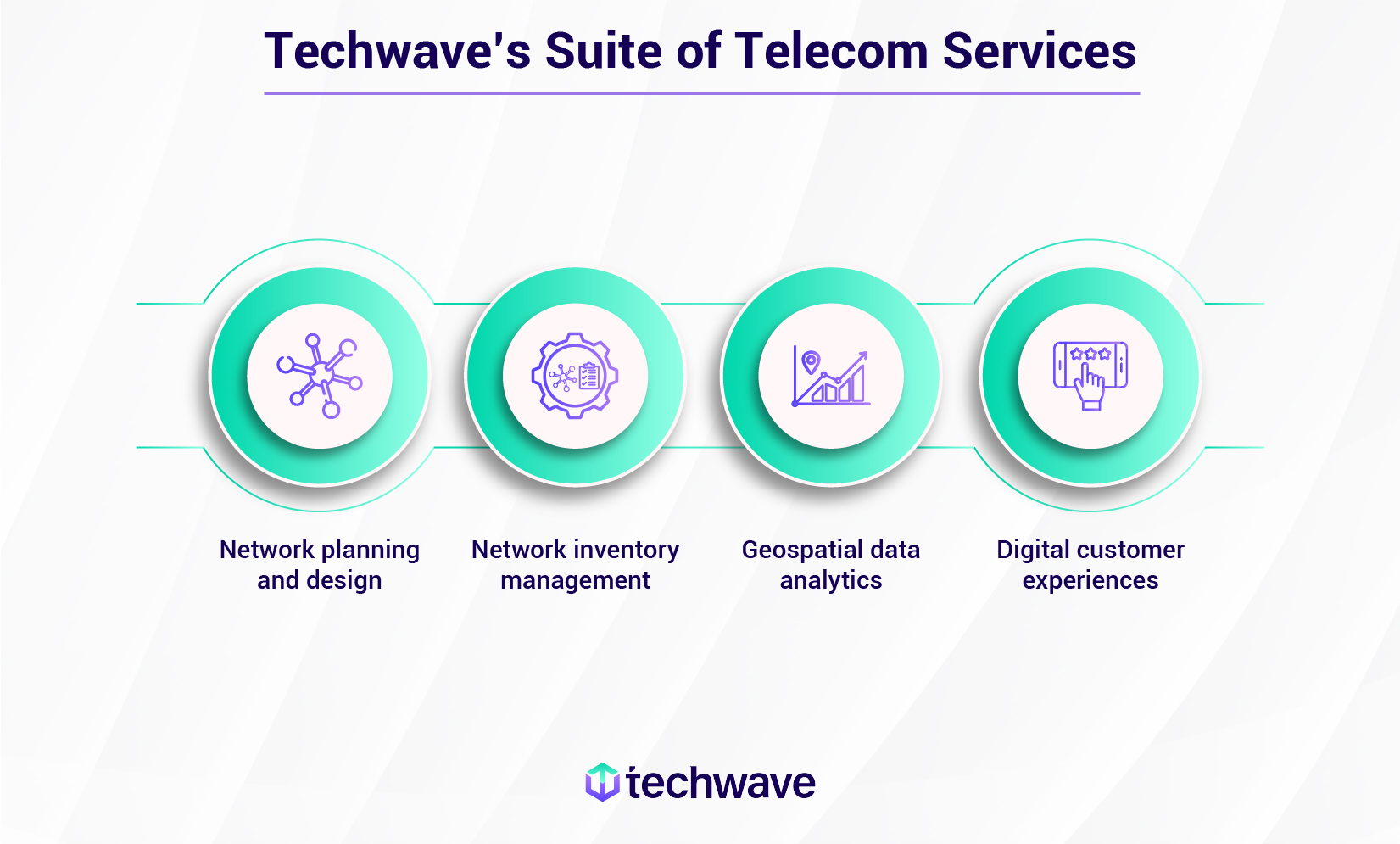
If you’re a telecom provider and the benefits outlined in this blog resonate with your goals, contact Techwave today to discover how our solutions can drive your business forward.