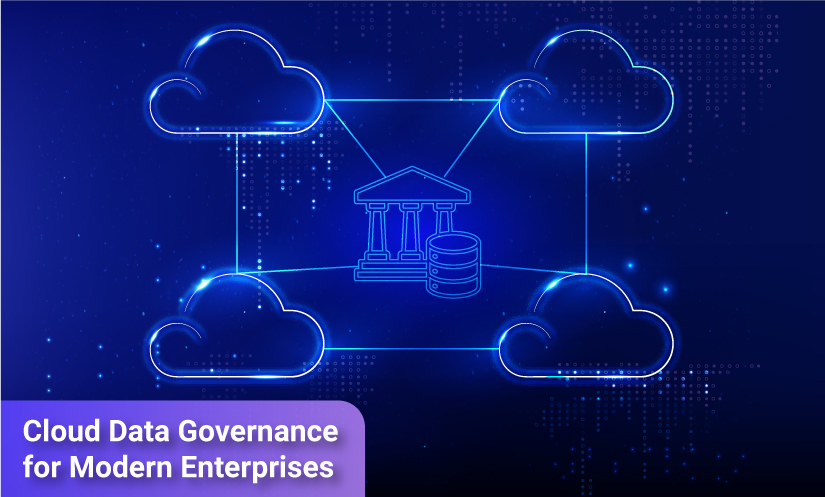
Many organizations assume that data alone can solve their decision-making challenges. Data is just the raw material to generate transformative insights for a business. In other words – the quality, accessibility, reliability, security, and relevance of the data and the advanced tools to weave them into insightful outputs truly drive the success of decisions.
The widespread adoption of cloud platforms and the surge in data have further emphasized the need for seamless cloud data management solutions. Today, envisioning a data-driven company without cloud services has become unthinkable. By 2027, Gartner predicts that 35% of data center infrastructure will be administered from a cloud-based control platform, a significant rise from the less than 10% recorded in 2022.
However, given its distributed nature, cloud data access has become easier, while managing and utilizing the dispersed data has become chaotic. Multi-cloud further exaggerates these challenges owing to its unique security capabilities and varying features across cloud providers. Consider a scenario where an organization uses a multi-cloud approach to enhance vendor resiliency. If one cloud encounters a DDoS attack, transferring the impacted services to another cloud is possible.
So, the question arises – How can we effectively leverage the distributed cloud data to create valuable insights? Cloud data governance is the answer.
What is Cloud Data Governance?
Cloud Data Governance (DG) dictates how an organization handles the security, accuracy, usefulness, and accessibility of its entire data infrastructure. This empowers an organization to guarantee dependable and credible data and insights. Therefore, cloud data governance-
- Ensures that cloud data assets are accessible to the authorized individuals for the right objectives, optimizing their security, quality, and value
- Aids businesses in understanding the data’s origin, nature, authorized accessibility, and appropriate retirement timelines
Evolving Cloud Data Governance – A Need to Modernize Your Cloud Data Governance Strategy
Employing a solid cloud data governance strategy ensures that your data is:
- Thoroughly audited
- Assessed
- Documented
- Handled
- Secured
- Reliable
However, according to Gartner, by 2025, 80% of organizations aiming to expand their digital business will only succeed if they adopt modern data governance frameworks in the cloud.
Why? Because existing governance strategies may not be able to match the pace with evolving cloud data technologies. As you may have noticed, despite advanced self-service analytics, cloud computing, and data visualization, governance remains a challenge! Following are a few reasons for the same:
- The modern cloud architecture generates real-time insights and enables easy data access but may fail to establish effective data democracy.
- Although data catalogs are touted as solutions for cloud data governance, many leaders find them deficient in basic manual requirements like thorough data documentation and metadata management and end up manually mapping dependencies between data sources, which requires ongoing maintenance to stay current.
- Data infrastructure and business intelligence tools may have evolved to support data innovation; however, dataOps has yet to catch up. Most dataOps solutions , such as data quality alerts and lineage tracking, are manual, limited, and lack scalability.
This implies that cloud data governance is akin to fitness: it’s not just a goal but an ongoing process—a lifestyle. Organizations cannot establish data governance policies and then neglect them, expecting continuous benefits. It must evolve to match the pace of innovation and relevance.
Modernizing Cloud Data Governance – 7 Common Obstacles Organizations Face
Following are some persistent cloud data governance challenges businesses must navigate during its implementation.
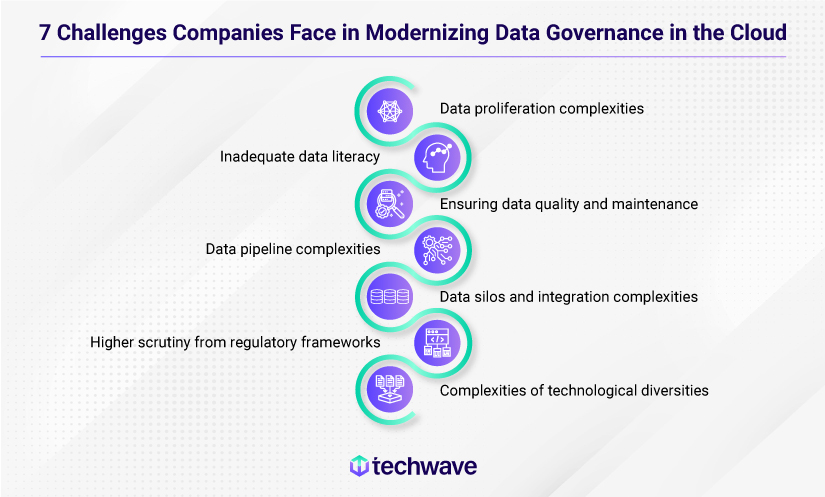
Increased complexity due to data proliferation
Cloud data warehouses stand out for their immense scalability, as they can handle virtually limitless data. These advancements enable organizations across industries to accumulate vast amounts of diverse and widespread data, characterized by the “3 Vs” of big data: substantial volume, variety, and velocity.
According to Forbes, 2.5 quintillion bytes of data are produced each day, which is truly staggering. This evolution necessitates an adaptive cloud data governance model to manage the booming data landscape effectively.
Inadequate data literacy
Tableau and Forrester surveyed 2,000 leaders and employees across 10 countries. The research revealed that although 82% of leaders anticipate all employees to possess fundamental data literacy, only 47% claim to have received data training from their employers. Moreover, only 26% of basic skills training is implemented company-wide.
Data professionals need a uniform comprehension of data across the organization and should be able to effectively leverage data to generate business value.
Difficulty in managing data quality and maintaining its standards
Data quality within cloud data governance essentially signifies the excellence of the data in use. High-quality data is accurate, complete, consistent, relevant, and timely.
Maintaining data quality is vital for sound decision-making, yet achieving this in the cloud can be challenging. Data sourced from various systems, platforms, and sources in the cloud might contain errors, duplications, or inconsistencies.
In March 2022, investment banking giant Credit Suisse faced a costly data quality error that resulted in a series of incorrect margin calls issued to hedge fund clients. This incident stemmed from a software bug that miscalculated the required margin for certain complex financial derivatives, leading to inaccurate data output. This example serves as a stark reminder of the domino effect that data quality issues can have within the interconnected world of finance. Even a seemingly minor error in a single institution can trigger widespread disruption and financial losses.
Increased complexities of pipelines and ecosystems
Before the cloud, data pipelines were basic, serving stable requirements for business analytics. Unlike the norm in early data warehousing, data movement has expanded far beyond the basic and sequential batch ETL.
- Managing multiple cloud computing environments adds to the growing pressure on businesses due to difficulties in scaling the network.
- Pipelines consist of processors and connectors facilitating data transport and accessibility between locations. However, as the number of processors and connectors multiply from various data integrations, it introduces design complexity, reducing ease of implementation and complicating pipeline management.
- Complex data pipelines incorporate data governance tools such as Apache Spark, Kubernetes, and Apache Airflow. However, their integration heightens the risk of pipeline failure due to increased interconnectedness. While these tools provide flexibility in choosing suitable platforms, they may impede visibility across pipeline segments.
Complex data pipelines swiftly become chaotic with the pressures of agile, self-service, and segmented analytics areas within an organization. Therefore, conventional data governance models struggle to oversee these complex systems, leading to unexplained data breakdowns.
Data silos and lack of data integration
Data silos hinder the accessibility and utilization of data across the organization. It can lead to fragmented insights, data duplication, and inconsistencies in decision-making.
For example, a single backup solution might demand dedicated components like backup software, master and media servers, target storage, deduplication appliances, and cloud gateways. These components can hold copies of the same data source. They often come from different vendors with unique interfaces and support contracts. This complexity results in multiple configurations, sometimes four or more, to back up different data sources of the same dataset!
These segmented silos impede operational efficiency. They restrict data sharing, causing excessive storage allocation for each silo instead of a shared pool. Furthermore, multiple data duplicates exist across these silos, needlessly using up storage space.
Specific challenges arising from data silos include:
- Rapid data accumulation
- Communication obstacles
- Preservation of legacy data in outdated systems
- Complicates integration of new data sources due to limited accessibility, duplication, and inconsistencies
- Various teams use disconnected applications and systems (e.g., marketing, sales, finance, and engineering).
- Non-progressive corporate culture
Given the lack of centralized governance across their environment, teams avoid sharing data between business units, which further reinforces silos. Consequently, IT teams must individually safeguard each data repository and, in the process, create their repositories (shadow IT) and amplify complexity.
Data currently faces greater scrutiny than before
As new privacy regulations emerge, managing data compliance remains a persistent challenge for businesses that can no longer afford to overlook it amidst heightened global scrutiny. According to Gartner, by 2024, 75% of the global population will have their personal data covered under privacy regulations.
Initially, data privacy regulations were restricted in scope. However, with the implementation of comprehensive frameworks like GDPR and HIPAA, global attention shifted significantly towards data privacy. As cloud technology progressed, these frameworks demanded compliance from all companies, creating challenges for businesses facing enterprise-level issues without affordable tools.
Technological diversity in data-driven environments
Technological advancements offer diverse tools for solving unique problems, yet their introduction often creates new challenges.
For example, a plethora of tools within data lakes for data ingestion, storage, processing, and analysis have led to integration challenges. These tools may come from different vendors or are developed separately. The differences in data formats, structures, and compatibility among tools within the data lake ecosystem may lead to compatibility issues when integrating them, especially in the absence of adequate governance, resulting in data chaos.
Adapting Modern Data Governance Practices to Cloud Environments
Cloud data governance best practices may vary across companies based on their specific data requirements. However, irrespective of your organization’s scale or sector, adopting the following governance practices is essential to maximize the potential of your cloud data infrastructure and insights.
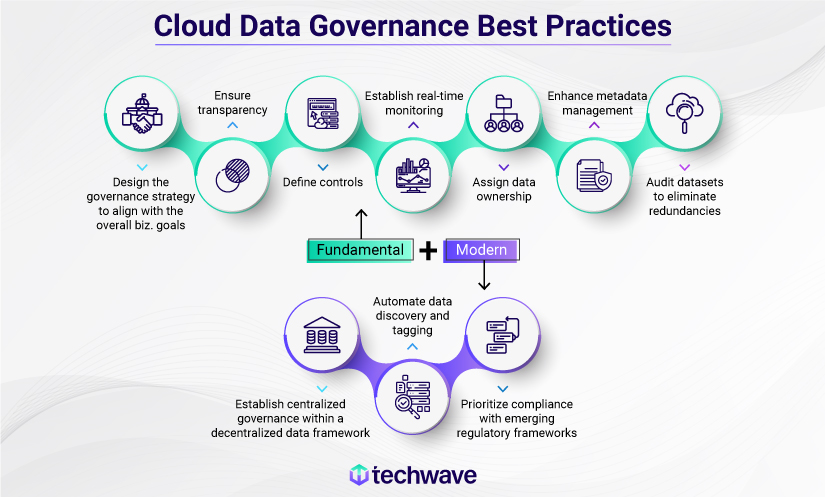
- Create a business case for the cloud data governance program emphasizing productivity, efficiency, cost savings, risk mitigation, and compliance.
- Ensure transparency among cloud data governance processes.
- Define controls after identifying your data domains.
- Establish real-time monitoring to maintain a smooth flow of high-quality data.
- Assign ownership to ensure efficient operation of the cloud data governance framework.
- Enhance metadata management by implementing consistent data management policies organization-wide.
- Track and audit replica datasets periodically to eliminate redundancies across various cloud systems.
While the above-mentioned fundamental guidelines can ensure an effective cloud data governance framework, the following best practices are specifically tailored to modernize your cloud data governance framework and align with evolving cloud needs and technologies.
Centralized data governance within a decentralized environment
Traditional architectures relied on centralized repositories managed by specialized teams for various business units. Modern organizations prioritize multiple domain-focused structures, departing from monolithic approaches to target specific growth areas.
Needless to say, cloud data governance must adopt a centralized control in a decentralized structure, illustrated by the data mesh model. Here, a centralized architecture is divided into smaller domain-specific (decentralized) “meshes,” managed independently by cross-functional “domain teams,” each responsible for handling data operations within their assigned domains. While it doesn’t enforce centralized control, a data mesh advocates centralized governance through standardized principles, practices, and data infrastructure.
For example, the various departments (sales, finance, HR, IT, etc.) can easily access their respective domains while also harnessing data mesh capabilities to get an accurate cross-departmental view of desired parameters. How? Data mesh ensures a set of centrally managed guidelines and standards determining how their domain data will be categorized, discovered, accessed, and managed. This enables a high degree of interoperability, implying a robust layer of standards that facilitates all the above alongside security and compliance. In simple words, centralized governance within a decentralized environment!
Automate discovery and tagging of data
Cloud-based environments offer an economical option for creating and managing data lakes, but the risk of ungoverned migration of data assets looms large. This risk involves the potential loss of knowledge about data assets in the lake, information within each object, and their origins. Cloud data governance and security platforms can address this concern by automatically identifying, categorizing, and tagging sensitive data across various platforms.
Effective data governance in the cloud enables data discovery and assessment to understand available data assets. This process involves identifying and documenting data assets within the cloud, tracking their origins, lineage, transformations, and metadata like creator details, object size, structure, and update timestamps.
For example, ‘Amazon Macie’ assists businesses in discovering and safeguarding their sensitive data on a large scale. This automation reduces manual classification and associated risks, applying access control policies automatically upon detecting sensitive data.
Data tagging is highly beneficial for Chief Data Officers (CDOs) to create policies that serve as rules or algorithms. These rules identify tags and apply specific regulations to any data carrying those tags. For instance, data tagged as “PHI” can automatically be stored in a designated location or restricted from specific uses to comply with HIPAA requirements.
Similarly, labeling all private health information data as “Protected Health Information (PHI)” streamlines future identification of its storage location, usage, and the applied data governance policies.
Prioritize compliance
Prioritizing compliance with crucial data security standards like FedRAMP, HIPAA, and ISMAP is essential for effective multi-cloud governance. It enhances credibility within the industry and simplifies safeguarding client and company data.
Cloud data governance strategy should clearly outline the responsible cloud governance tools, processes, and individuals for acquiring and maintaining compliance. Labeling data based on sensitivity ensures relevant security protocols. Additionally, data protection aligns with global regulations like the GDPR and CPRA.
Techwave’s Cloud Data Governance Services
Techwave assists organizations in establishing guidelines, protocols, and criteria for overseeing data assets, ensuring adherence to these established policies. Our partnership with esteemed technology brands such as Amazon, Google Cloud, Tableau, and many others positions us as the ideal partner for advanced Data & Analytics solutions that span data and cloud governance. Techwave’s cloud governance services offer secure cloud data management, fostering trust in providing exceptionally satisfying services.
Please get in touch with us to learn more about our services and how we can help you implement cutting-edge cloud data governance and maximize ROI from your cloud investments.